Proposal to Make Full Use of Data from Web Inspection System by IoT Technology
- Auto Web Inspection System
- Quality Control
- BI
- Condition Based maintenance
- Internet of Things
Recently, manufacturers have some common issues such as highly developed manufacturing, complicated manufacturing, or manufacturing with few skilled people. They are trying to solve these issues by IoT technologies based on big-data which are corrected from the machines in the manufacturing site.
OMRON Corporation Inspection Systems Business Division has been providing the auto web inspection systems which have Multi-wave sensing technology to find defect by utilized several light sources which have different wave length.
In this report, we have developed brand-new service named 窶廴ONOSASHi窶 service, based on IoT technology. The service can visualize various manufacturing condition to improve productivity using our auto web inspection systems.
1. Introduction
Manufacturers have to pursue value-added manufacturing with high quality and stable operation. Now they also have to adapt to global competitions and to developments in new technologies1シ. In the recent environment surrounding the manufacturing sector, the Industrie 4.0 in Germany and the Industrial Internet Consortium in the United States2シ have triggered a trend toward technological innovations that would change the whole scenery of manufacturing shop floors. Among such innovations are remarkable advancements in Internet-of-Things (IoT) technology in addition to robotics technology and artificial intelligence (AI) technology. In Japan, after the establishment of conferences, such as the Industrial Value Chain Initiative3シ, manufacturers are getting ready to support robots, IoT, and computerization. Now manufacturers are expected and required to be flexible enough to adapt to major shifts. Examples of major shifts may include satisfaction of individual needs through multi-product, small-lot production; sophistication and complication of manufacturing due to the developments in data-based factory management; firm establishment of global production systems based on multipolar production; and increasing needs for automation due to changes in the job market such as skilled personnel shortage or soaring labor costs. In other words, to keep up with evolutions in manufacturing or with changes in market needs, it is becoming more and more important to make effective use of constantly changing cutting-edge technologies.
To serve such manufacturing needs, our Inspection System Division sells inspection systems equipped with cameras and has in its product range visual inspection systems (AOI) for inspecting solder joints and parts mounted on printed circuit boards4シ, CT X-ray inspection systems4シ, as well as Web inspection systems for performing high-speed, highaccuracy inspection for surface defects on high-functional films or plain webs used for secondary batteries or displays5シ (Fig. 1).
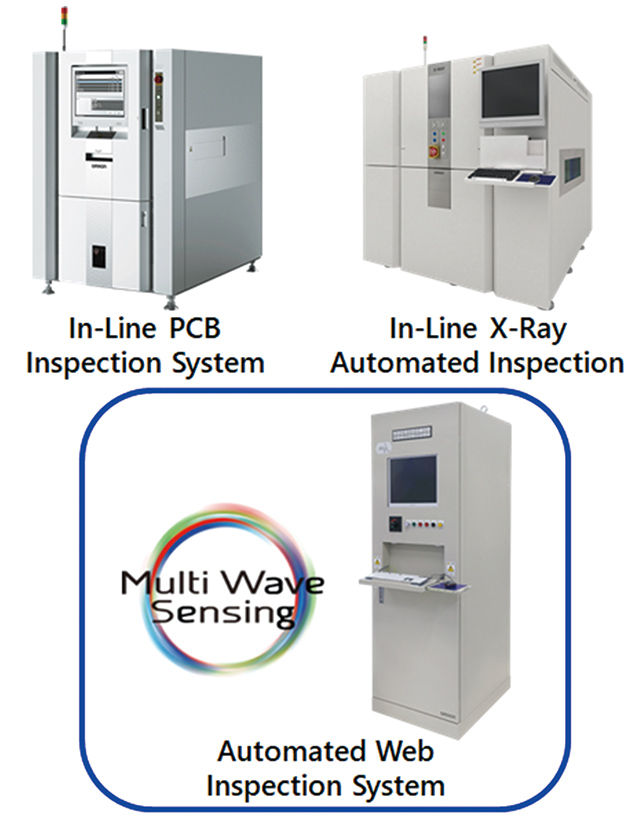
This paper places focus on Web inspection systems and proposes OMRON-proprietary new services (MONOSASHi Services), which not only make these systems available as simple defect determination systems but also apply IoT technology thereto to guide users to shop-floor improvements including monitoring of stable operation of inspection systems, validation of inspections, and product quality improvement.
2. Challenges
Typically, an OMRON-made Web inspection system has more than one light source, such as near infrared and ultraviolet, from which the optimal wavelength light for inspection is selected and radiated to the object under inspection. This system is based on multi-wave sensing technology, which achieves high detection power and high determination performance by in-line cameras capturing the as-is images of objects under inspection and by a dedicated algorithm processing these images. In this way, the inspection system performs inspection by detecting and classifying normal regions (formations) and anomalous regions (defects) in the object under inspection (Fig. 2).
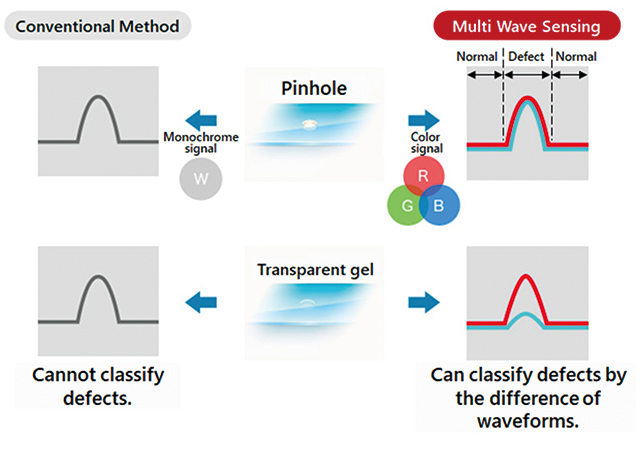
As shown in Fig. 3, the inspection results obtained from the system are displayed on the screen consisting of a defect list containing a full list of defects, a defect map showing the locations of defect occurrences detected during the inspection of one full lot, and a frame containing defect images accompanying the defect list and defect map. These pieces of output information contain mainly defect-related information, such as feature quantities characterizing defects.
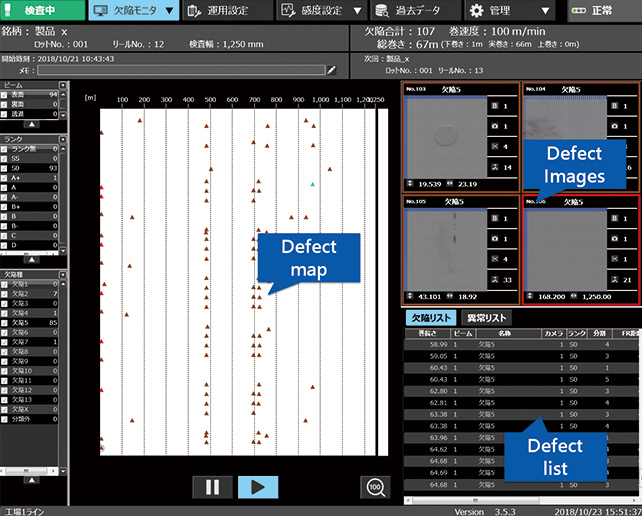
These pieces of information are, however, output information produced per roll of product as product inspection results showing only what kinds of defects occurred when and where. Thus, no additional pieces of information are available, such as why such defects occurred or whether there were any precursory signs. To detect precursory signs, time series must be examined for changes. None of our current systems provides more than pass/fail information on a roll-by-roll basis. To examine more detailed data in a time series, the user must compile source data manually. To make the matter even worse, neither are they equipped with a function for managing fluctuations of the intra-roll product condition. As a result, our current systems have ended up with information extremely inconvenient for users to apply to their shop-floor improvement activities. Based on the above observations, the author considers it necessary not only to improve the current use of fragmentary information from the inspection results but also to solve the following challenges in order to apply Web inspection systems to usersハシ shop-floor improvement activities and achieve advances in manufacturing:
- (1) How to compile and collectively manage on a time-series basis quality information available from more than one inspection system
- (2) How to display the normal condition (conforming product condition) quantitatively based on collectively managed information and validate the inspection
- (3) How to change on-screen viewing methods and displayed information flexibly according to shop-floor improvement activities differing from one user to another
3. Solutions for effective use of inspection system data
Fig. 4 shows a typical high-functional film manufacturing process. The Web inspection system is positioned at the final step of the manufacturing process. Hence, the quality information available from the inspection system is expected to vary depending on the manufacturing process. Information available from the Web inspection system may include conforming product information, defect information, information on the system itself, and, if need be, information on user operations and other events. The author then hypothetically assumed that such a collection of data can used not only for the Web inspection system but also for identification of problematic factors associated with the manufacturing process or for manufacturing line condition diagnosis, including predictive management, thereby making it possible to make effective use of inspection system data for usersハシ production activities and quality improvement activities.
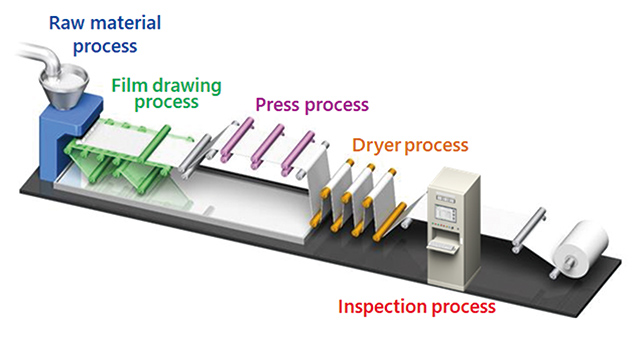
3.1縲Compilation of quality information
To make effective use of such new quality information available from a Web inspection system, first, all the available quality information must be collectively consolidated simultaneously whether or not there is an inspection in progress. This is where IoT technology should be applied. More specifically, the Web inspection system so far used stands alone separately from the network and will be connected to the local area network (LAN). This will allow quality information from more than one inspection system to be collectively managed as big data without affecting inspections. Through analysis of such big data, quality information will become available to diagnose the condition of more than one production line. In future, interfactory production line diagnosis will become possible through the use of cloud computing services.
3.2縲Visualization of inspection validation results
In the current inspection process, a Web inspection system is used exclusively to detect defects, which are anomalous portions of the product. Hence, data on conforming portions are not used for the most part. If, however, data on conforming portions and those on defective portions, together with inspection threshold information, could be collected and visualized, it would then be possible to view how much the conforming and defective portions differed from each other. In addition, comparisons with inspection thresholds would show objectively and quantitatively whether inspections had been performed properly.
In multi-product, small-lot production, for example, lot-by-lot comparison would show whether the production had been performed the same as the last time, including conforming portions. Moreover, at the start-up of a production line for a new model, it would become possible to visually determine an optimal inspection threshold by comparing conforming products with defective products of detection interest in terms of the degree of variation.
So far, an on-screen defect map has been used to show defect occurrence locations and defect occurrence trends on a lot-bylot basis. A stretched version of this map with coverage over more than one lot, if used in combination with statistical information based on defect occurrence locations, would help to identify the process or processes responsible for the defects.
Furthermore, the effective use of information from inspection systems would make it easier to establish firm global production systems, launch new brands, and perform improvement activities based on quantitative analytical determination rather than based on intuition, talent, or experience. The author expects that defect trend management for each causative factor through the effective use of AI technology will facilitate identification or predictive management of the causative factors in the manufacturing processes, whereby our Web inspection systems will be upgraded into process monitoring systems able to provide process control information resistant to variations in proficiency among operators.
3.3縲Flexible user interface
To handle as big data the stock of data collected and stored as described above and effectively use it as the clue for solving the challenges in usersハシ improvement activities, the following visualization functions will be necessary:
-
(1) Analysis and visualization of production information
In addition to a lot-by-lot defect list and a map function showing defect locations, a function that analyzes and visualizes the locations of defect occurrences, as well as their frequency and severity, without restricting them within lots on a daily, monthly, and annual basis -
(2) Continuous visualization of the state of the conforming product
A visualization function that monitors the inspection status with or without any defect occurring and shows the degree of conformity and the as-produced state of the product -
(3) Visualization of the maintenance status and hardware information
A visualization function that enables implementation of necessary maintenance at the optimal timing in addition to periodic judgments on, for example, the replacement time specified for each piece of hardware
Such visualized information, however, depends on the nature of the userハシs manufacturing process or improvement activity. Its most effective form would differ from one user to another. Hence, the user interface must allow flexible adjustments. So far, typical inspection systems have been supplied standard with a fixed built-in user interface. Such a user interface requires user-specific customization, and as required by each user, the detailed design must be taken back to the drawing board to start all over again from the specification review, thereby resulting in considerably increased lead-time and costs. The author proposes the use of Business Intelligence (BI) Tools, one of the latest IoT technologies, as a solution to this problem. BI Toolsハシ advantages, namely, flexible visualization and data analysis functions, make it easier to perform user-specific customization of the user interface. As a result, the user interface will become able to provide necessary user-specific add-ins and graphic representations quickly and timely.
4. Methods for implementing and providing solutions
4.1縲Implementation method
Fig. 5 shows the system configuration the author proposes. As shown in this figure, each Web inspection system will be connected to LAN. Then, an integrated management server will be installed that collects big data and performs data storage, analysis, and visualization functions. This will enable collection of different types of data from more than one inspection system in the same factory. The term LAN here means a local area network that extends from the integrated management server and does not necessarily have to be connected to an in-house LAN or an external network. The integrated management server will regularly access each of the inspection systems via the LAN and collect big data including not only inspection results data but also system information data.
The big data thus collected will be a collection of miscellaneous data. Hence, the as-is storage will result in a huge amount of time required for data analysis and visualization. Hence, the author has chosen to extract valuable data from this collection of big data and to edit and store them in forms easier for analysis and visualization. Then, BI Tools will be used to analyze and visualize the big data. The screens to be displayed will be provided as template forms. Then, filledout on-screen verification forms will become accessible from a Web browser. In future, therefore, on-cloud operation of such template forms may enable effective use of Web inspection systems without time and location restrictions.
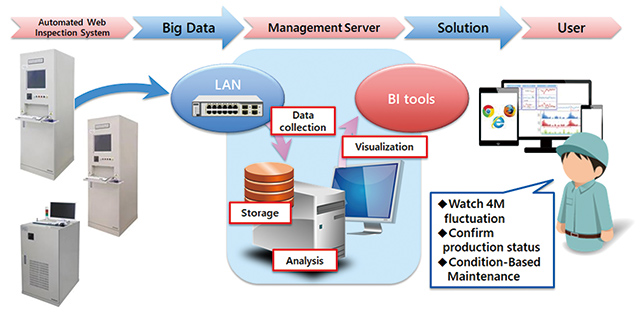
4.2縲Provision method
As explained in Section 3.3, challenges to be solved in shopfloor improvement activities may differ among users and vary depending on their respective methods of analysis and verification. Solutions to these challenges, if built into a conventional single standard system, would have to take the form of applications that provide all UI skins and analysis techniques necessary for individual users. Consequently, the system might end up large and complicated and, in the worst case, might become an impediment to improvement activities. Accordingly, the IoT system we propose will be provided as add-ins with easily customizable screens, mainly from the following three perspectives:
-
(1) Inspection criteria development
Conventionally, when developing inspection criteria, a user has had to determine them based on actually observed or simulated defects. Unlike usually welldefined numerical criteria of the size of the defect, criteria difficult to define numerically, such as the brightness or color of defect, have had to be determined by the user based on their intuition, talent, and experience while looking at a defect image (Fig. 6).
Consequently, such inspection criteria have often ended up difficult to justify for appropriateness. To be provided as solutions to such challenges are add-ins that visualize conforming and defective product conditions in a time series. With conforming and defective product distributions visualized in a time series, the user will be able to easily find points to which to set inspection criteria based on fact data (Fig. 7).
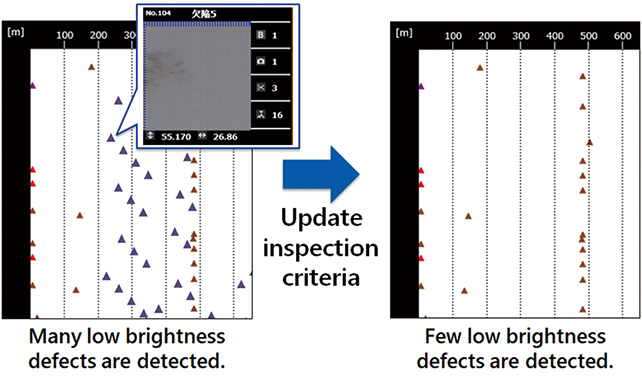
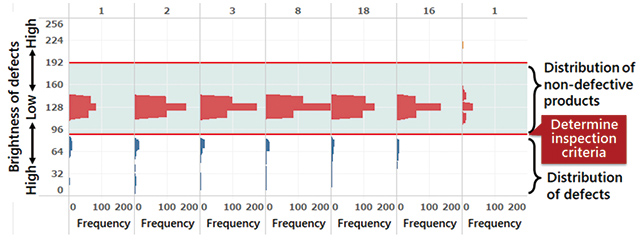
-
(2) 4M fluctuation monitoring
It is a common practice to perform shop-floor improvement activities based on observed fluctuations in inspection, namely, observed fluctuations in the 4M (man, machine, material, and method). Our conventional inspection systems are not equipped with a method of presenting the user with quality information in time series. Hence, when required to view fluctuations in the 4M, the user has to perform a manual comparison of quality information or develop verification tools. As a solution to such a challenge, product quality information should be visualized in time series, using both defective and conforming product information, to allow an easy grasp of product quality status that gives the basis for verification of fluctuations in the 4M. -
(3) Condition-based maintenance (CBM)
In the case of an inspection system, time- or intervalbased maintenance is often performed as part of hardware condition monitoring. This maintenance takes place at time intervals similarly to periodic inspections. The challenge to this maintenance boils down to timing. Too short an interval may result in over-maintenance. Conversely, too long an interval may result in failure before the next maintenance. Thus, the right timing to perform necessary maintenance actually remains unknown. This explains why unnecessary maintenance takes place or why necessary maintenance cannot be performed at the time of periodic maintenance (Fig. 8).
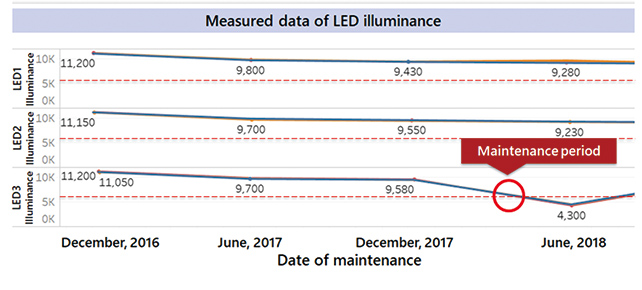
As a solution to such a challenge, the degree of deterioration of parts in the inspection system should be continuously monitored to allow maintenance of the necessary parts and proper operation of the inspection system. If these add-ins are provided as a form of MONOSASHi Services to users, the proposal and provision will become possible of user-specific optimal methods of data presentation and analysis. In addition, connections can be established to Web inspection systems already in operation to provide services thereto. This allows collective management beyond generations of inspection systems. In this way, Web inspection systems can contribute to defect-free manufacturing traceable to conforming products.
5. Effect verification
This chapter presents several cases where MONOSASHi Services were applied to Web inspection systems in operation on customersハシ manufacturing lines and were observed to contribute to shop-floor improvement activities.
5.1縲Inspection criteria development
This section presents a case where an inspection criteria development add-in was employed to propose to a user a recommended value for an inspection criterion based on their inspection status. Fig. 9 shows the add-in provided to the user. As shown by the Formation Trend Summary in Fig. 9-(1), data on conforming portions (formation data) and on defective portions were periodically obtained to visualize their distributions in a histogram on a roll-by-roll basis. The time series shows that the fluctuations in conforming portions (red) remained stable and fell within the inspection criterion. On the other hand, the fluctuations in defective portions (blue) were close to the inspection criterion. Thus, the time series revealed that the then-current inspection criterion was posing a risk of occurrence of undetected and overlooked defects. Then, the overall histogram distribution and the relevant rollハシs histogram distribution (overall distribution and intra-roll distribution in Fig. 9-(2)) were studied in detail to find that the end of the distribution of conforming portions was separate from that of defective portions and hence that a new inspection criterion could be established in between the former and the latter. Based on this finding, we proposed to the user a median value as a recommended value. As a result, the user successfully prevented defects from passing undetected and overlooked. Thus, the user can grasp at a glance the relationship of the fluctuations in conforming portions to the inspection criterion. The foregoing observation confirms that the user can specify or modify inspection criteria immediately based on fact data displayed as histograms.
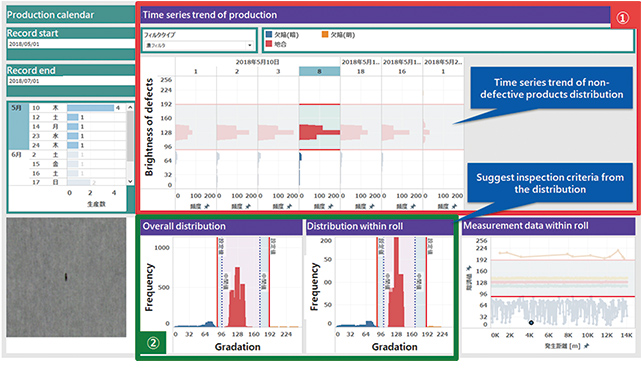
5.2縲4M fluctuation monitoring for checking the condition of the manufacturing process
This section presents a case that became the starting point of improvement when a change in the condition of the product manufacturing process was detected during the daily monitoring of the condition of the product manufacturing process using an add-in intended for the 4M fluctuation monitoring. As shown in Fig. 10 (1), product quality information was automatically collected and converted into a time-series graph with information necessary for the user. In this way, the day-today work so far performed manually by the user to check the condition of the manufacturing process was eliminated along with the need to develop a dedicated system. Moreover, a key performance indicator (KPI) was set as a product quality indicator. This motivated the user toward indicator-based product quality checks and improvement activities.
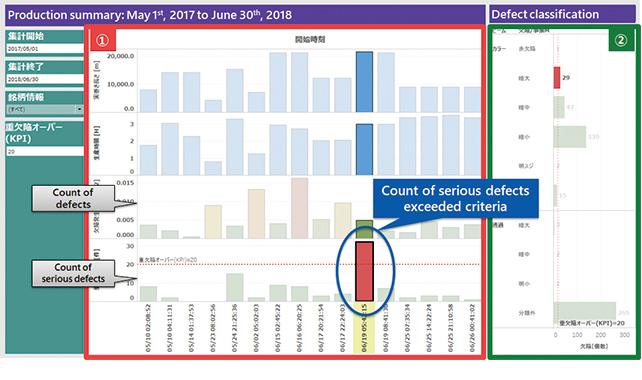
Following the detection of a manufactured roll containing a greater number of serious defects (quality information) than the KPI, a detailed, in-depth analysis was performed of the inspection results. First, the Inspection Results Viewer was opened to check all inspection results (Fig. 11).
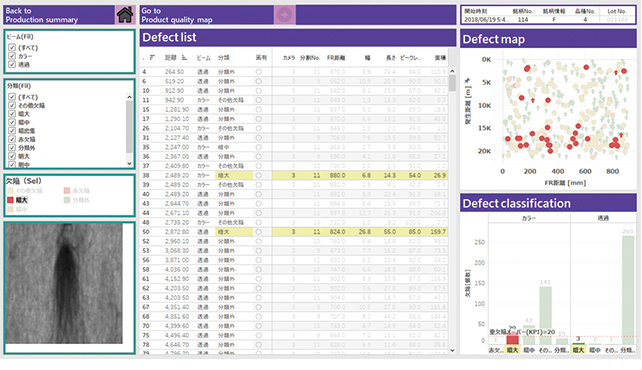
When defects violating the KPI were highlighted, the defect map revealed that the relevant defects occurred in a locally concentrated manner. Then, an add-in designed to check for an uneven distribution or a characteristic trend in defect occurrences was used for further analysis. Fig. 12 shows the add-in used for this analysis. The analysis found that serious defects occurred densely near specific points in the Web flow direction along the length of the roll while defects did not occur unevenly in the width direction (in-line camera view direction) perpendicular to the Web flow direction. The above observations showed that the add-in was effectively used to motivate the user toward improvement activities for monitoring the occurrence of sudden events during the manufacturing process and to help the user grasp the current state as the basis for necessary improvements.
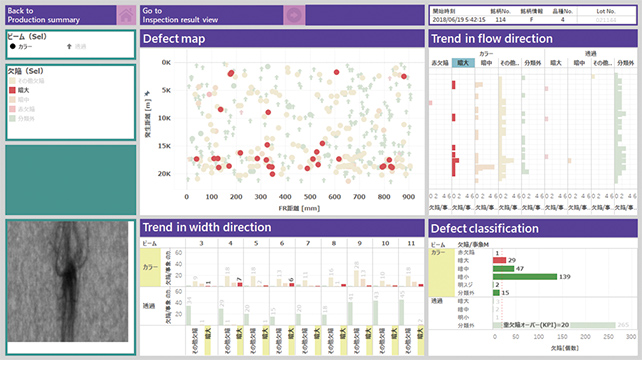
Thus, it was confirmed that the add-in developed and improved as a BI Tool to suit the event of the userハシs analysis interest allowed effective use of Web inspection system data for shop-floor improvement activities.
5.3縲Camera gain monitoring for evaluating the deterioration of LED light sources
This section presents a case where a condition-based maintenance (CBM) add-in was used to evaluate the deterioration of LED light sources. The LED light sources in a Web inspection system are parts of critical importance for inspection. Hence, the monitoring of the deterioration of LED light sources is indispensable to ensure stable operation of an inspection system. The user can evaluate the deterioration of an LED light source by measuring the quantity of light from the LED using an actinometer. In a high-functional film manufacturing process running 24 hours a day, 365 days a year, however, there is always a film under inspection on the inspection surface. Therefore, the user can measure the quantities of light from the LED light sources only at the time of periodic maintenance during which there is no film on the inspection surface. On the other hand, to perform inspections normally, the Web inspection system controls the camera gain to suit the brightness of the item under inspection, whereby the brightness of the item under inspection is kept at a constant level. Accordingly, when the quantity of light from an LED light source decreases and reduces the brightness of the item under inspection, the camera gain is expected to increase. Therefore, the degree of deterioration of LED light sources can be predicted based on the fluctuations or rising trends in the gains of cameras under continuous monitoring. This led the author to the idea of applying the theory of CBM devised to enable optimally timed maintenance.
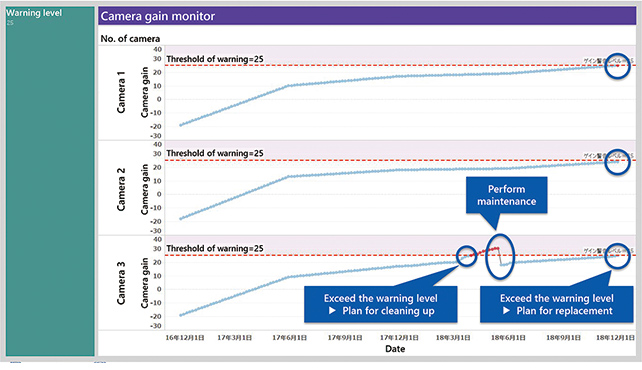
When a warning level is exceeded, as in Fig. 13, during periodic camera gain monitoring, a prompt message can be issued to urge the user to plan for preventive cleaning and maintenance. The author expects that the combination of maintenance thus timed and a continuous results monitoring using this add-in will reveal the effect of the maintenance, thereby assuring continuous stable operation of the inspection system. At present, the author has yet to verify the expected effect because LED light sources deteriorate very slowly. Fig. 13 merely shows a theoretical hypothesis partly based on the currently available observation data. The author will continue observation until the effect is verified.
6. Conclusion
This paper has proposed a new approach to effective use of quality information data available from Web inspection systems through IoT technologies to suit any changes in the recent environment surrounding the manufacturing sector. We intend to contribute to production activities and quality improvement activities as a Web inspection system manufacturer. For this purpose, we will continue developing cutting-edge technologybased Web inspection systems and effective applications thereof to keep up with evolutions in manufacturing and changes in market needs.
References
- 1シ
- OMRON Corporation. 窶廾MRONハシs view on Industrie 4.0窶. https://www.edge-link.omron.co.jp/news/99.html, (accessed 2019-2-1).
- 2シ
- Industrial Internet Consortium. https://www.iiconsortium.org, (accessed 2019-02-01).
- 3シ
- Industrial Value Chain Initiative. https://iv-i.org/wp/ja, (accessed 2019-02-01).
- 4シ
- OMRON Corporation. 窶弃CB inspection system窶. https://www.fa.omron.co.jp/product/special/pcb-inspection-system/, (accessed 2019-02-01).
- 5シ
- OMRON Corporation. 窶弩eb inspection system窶. https://www.fa.omron.co.jp/product/special/web-inspection-system/multi-wave-sensing/, (accessed 2019-02-01).
The names of products in the text may be trademarks of each company.