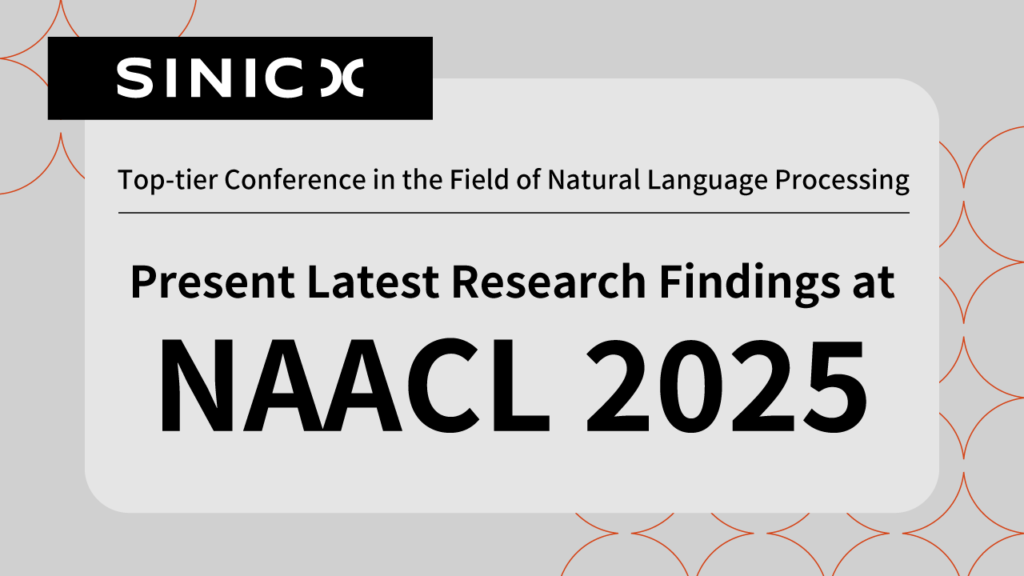
OMRON SINIC X Corporation (HQ: Bunkyo-ku, Tokyo; President and CEO: Masaki Suwa, hereinafter “OSX”) will present the latest research findings at 2025 Annual Conference of the Nations of the Americas Chapter of the Association for Computational Linguistics (hereinafter ” NAACL 2025″).
NAACL 2025 is one of the top-tier international conferences in the field of natural language processing. The conference will be held from April 29 to May 4, 2025, in New Mexico, United States (local time).
The research papers to be presented by OSX are as follows:
NAACL 2025 presentations
■ Where is the answer? An empirical study of positional bias for parametric knowledge extraction in language model
This paper investigates how language models memorize information and extract it in the form of questions. The language model performs self-supervised learning using unlabeled text data, during which it learns knowledge about various facts. This knowledge is required to be “extracted” from the model’s parameters to answer specific questions.
However, traditional methods face the issue known as the “perplexity curse1).” This refers to the phenomenon where, even if the loss is minimized for the training data documents and the model memorizes them accurately, it is difficult to extract correct answers to actual questions. This study investigates this problem and demonstrates that a “positional bias” in the training data is one of the causes of this issue. Specifically, it was found that the model struggles to answer information presented in the middle or end of sentences in the training data. The study shows that this problem is caused by autoregressive learning (the method where the next token is predicted based on previous tokens). These findings are considered to be key to effectively extracting knowledge from the language model’s parameters.
1) Zhengbao Jiang, Zhiqing Sun, Weijia Shi, Pedro Rodriguez, Chunting Zhou, Graham Neubig,Xi Victoria Lin, Wen-tau Yih, and Srinivasan Iyer. Instruction-tuned language models are better knowledge learners. arXiv preprint arXiv:2402.12847, 2024.
https://arxiv.org/abs/2402.12170
※Author information is current as of the date of writing or submission. Please be advised that the information may become outdated after that point.
For any inquiries about OSX, please contact us here.